
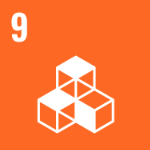
9. Industry, innovation and infrastructure
Data Science vs. Humans: who do we trust in the decision-making process?
There is no doubt that data science applications are becoming a part of organizations’ lives and, consequently, the lives of us all. From familiar aspects, such as product and content recommendations (think Facebook, Netflix, and Spotify, which analyze our characteristics and preferences to suggest the next product we may like); to more surprising applications such as one that suggests recipes based on the ingredients we have in our kitchen [1], artificial intelligence algorithms are pervasive in our daily lives.
Does this permeability to artificial intelligence means that, soon enough, we will let algorithms make the decisions that matter in our organizations and in our society? As technology itself evolves and becomes more effective in detecting patterns and preferences, should we let it tell us which direction to follow? Will data science algorithms make human intuition obsolete? Two main reasons may justify a negative answer to these questions:
1. Applying data science to human behaviors requires human subjectivity
Typically, artificial intelligence algorithms analyze vast volumes of data to find patterns associated with a particular group or class. For instance, we can train an algorithm to tell the difference between a dog picture and a cat picture, by providing enough photos of each category for it to learn the main characteristics of each animal.
It has been proven that these algorithms can, in fact, learn these patterns in a precise and effective way. And this is true also regarding problems as important as preventing car accidents [2] or identifying people on the verge of committing suicide [3][4].
It is precisely in sensitive human problems that it becomes clear that algorithms cannot have all the answers. On one hand, there may be people that do not fit the detected patterns and whose identification requires empathy and sensibility that only another human being can offer. On the other hand, while an algorithm may suggest which actions to take next, their validation and, ultimately, their implementation, must be made by people, with all the inherent subjectivity that human relations have.
And this idea not only applies to more extreme cases: for instance, managing the relationship with an unhappy customer requires sensibility that may complement or even defy the actions suggested by the algorithm.
However, this does not mean that data science algorithms must be discarded. On the contrary: they can be a fundamental tool to destroy preconceptions, suggest new paths, manage clients and identify cases that are invisible to the human “eye.” It underlines, however, that we should not hand over the entire decision-making power to an algorithm. It is also fundamental to leave room to more subjective decision-making factors.
2. The development process of data science algorithms integrates human subjectivity
Though sometimes we tend to look at data science algorithms as something completely objective, it is essential to recognize that they also have a subjective component, inherent to their own development. In fact, subjective human aspects are also an integrating part of the process of building data science models.
The quality of any data science model is determined by the quality of the question that originates it. In a simple example, asking who are the clients that will join a specific campaign is not the same as asking which campaign will make which clients more satisfied. Likewise, the decision of which data to collect also derives from subjective choices. “Garbage in, garbage out”: an algorithm can only offer good outputs if it is fed complete, adequate and non-biased data, something that is people’s responsibility to guarantee.
In conclusion…
Data science applications will be increasingly present in our day-to-day lives, informing and guiding the decision-making process in numerous areas. These applications can bring a contribution that goes beyond the human capacity to analyze and answer complex questions. However, it is crucial to not only understand data science merits but also their limitations — acknowledging the need to make room for subjectivity that (at least for now) only humans are capable of.